Our professional path mainly depends on our readiness for continuous development, active training, and exploration of new information and knowledge. To stand out in the job market, it’s crucial to be familiar with trends and anticipate and adapt to them.
In mechanical designer work, besides rapidly evolving production technologies, the use of artificial intelligence (AI) is a widely discussed and promising area for development. AI and ML (Machine Learning) have played important roles in the mechanical engineering sector for years.
Specifically in the role of a designer, it is used in:
- the automation of CAD/CAM operations,
- analysis and simulation,
- parameterization
- and many other functions about which a separate article could be written.
Without the involvement of artificial intelligence and machine learning, some simulations wouldn’t be possible.
However, the main aim of this article is to present new trends in the approach to designing with AI support, highlighting their innovative nature, often extending beyond standard perceptions. While you may feel that some of these options are familiar from previous versions of your CAD software, they are becoming increasingly advanced in the face of the rapid development of artificial intelligence, making their usefulness increase significantly.
If you have been using CAD routinely for a long time and are looking for new opportunities, here are some tools and new design approaches that may soon become commonplace.
Generative design
In traditional design, the designer transfers his idea to a computer model. One clear limitation is that they can only create a few simplified product concepts because of the required time. In addition, early-stage proposals usually come from a single person with specific design tendencies, limiting solutions’ variety and innovation.
Generative design is the reverse of this process – here, the designer specifies inputs such as:
- material type,
- weight,
- strength,
- manufacturing technologies or product price.
On this basis, the computer, using algorithms based on artificial intelligence, creates thousands of concepts and analyses each. This is one of the key steps in using the generative design function because if we wrongly define the boundary conditions, it is not difficult to get the wrong solution with this type of simulation.
Let’s take a look at General Motors’s results. In collaboration with Autodesk, General Motors created a seat bracket in which seat belts are fastened as part of the technology described. The program has created 150 innovative concepts that are decidedly different from the standard box-like form and more reminiscent of metal objects from outer space.
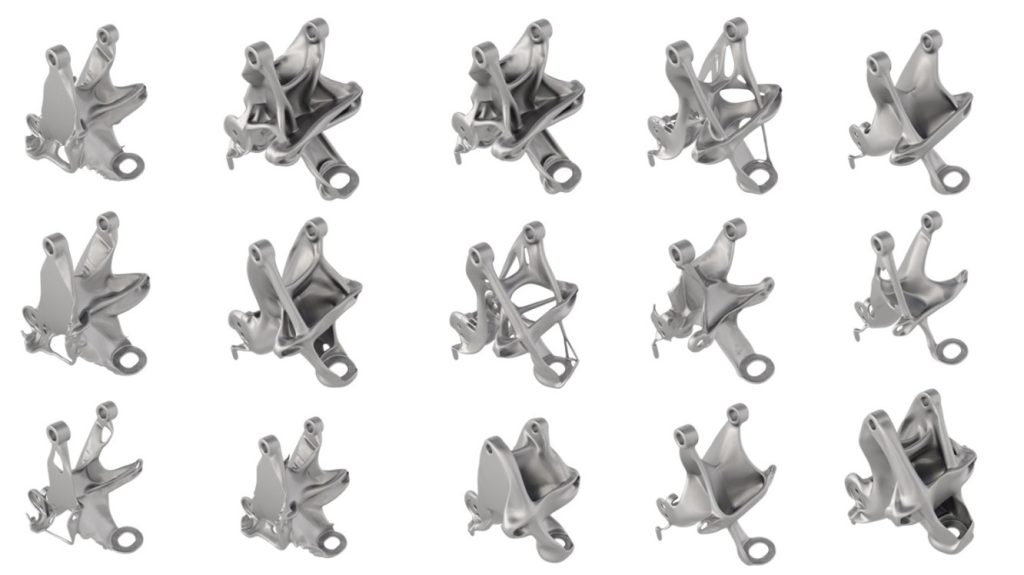
An unquestionable advantage of such a solution is the exploration of design space in a way that would be impossible to achieve using the human mind, creating elements with a futuristic shape.
The role of the designer
The next step is to verify the designer’s obtained concepts, select the most accurate model, and then refine and modify the existing assumptions.
The solution obtained this way is usually produced using 3D printing technology, which is often an integral part of the Generative Design process. The finished component has reduced weight while increasing strength, as the algorithm has been able to remove material where it is not needed.
Moreover, the designed structures can have specific aerodynamic or mechanical properties already in the project’s initial phase, reducing human labor and thus shortening the time needed for product development.
Another distinctive feature of the solutions obtained is the consolidation of parts. In the case of the General Motors seat bracket mentioned above, eight components were replaced by one. The part is 40% lighter and 20% stronger.
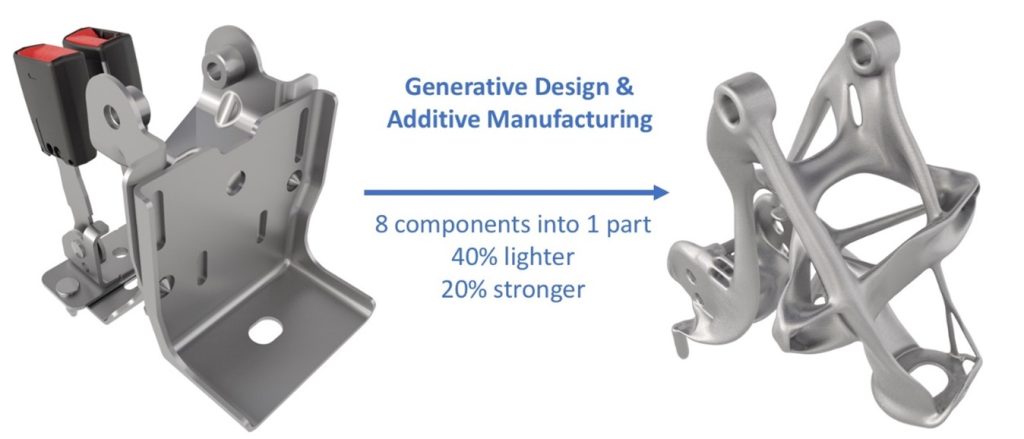
Combining generative design and incremental technology could completely transform the automotive manufacturing world. The industry has historically been constrained by the ability of machines to produce only simple geometries.
It also gives hope that designers will gain more time to focus on higher-value decisions, such as maximizing part performance while minimizing repetitive tasks.
Behavioral modeling
Another functionality useful during design is behavioral modeling, which uses neural algorithms to analyze the behavior of an existing model and predict its response to changes in parameters or conditions. To better understand this approach, consider the example of designing a belt drive.
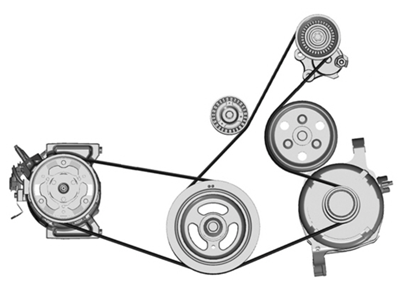
Such a process involves a series of steps that require consideration of various factors, such as:
- safety factor,
- power transmitted by the belt,
- shaft rotations,
- type and dimensions of belts,
- as well as determining mounting constraints.
In addition, the interaxial distance and other relevant parameters must be determined. Without the use of behavioral modeling, an engineer would have to rely on trial and error, conduct numerous analyses, or have extensive drive design experience to match standard components such as pulleys and belts to a specific drive design.
In the case of behavioral modeling, the process will consist of three stages:
- Entry of information and data (also in the form of a 3D model or analysis) into the system
- Testing the behavior of the model while modifying the environmental conditions
- Solving design problems
Once all the input data (loads, moments, stresses, drive output speed, and more) and some geometrical assumptions are prepared to observe the behavior, a simulation of how the model reacts to parameter changes is carried out. This results in multiple scenarios with different configurations of the input parameters.
In the case of a belt drive, the engineer can investigate how belt length or load changes affect its stability and performance. Analysis functions are created with behavioral modeling that automatically evaluates parameters for different configurations. Based on the results of these analyses, the most optimal solution that meets the performance and compactness requirements can be easily selected.
AI-Assisted Design – design supported by Artificial Intelligence
If artificial intelligence has supported design in each of the methods described so far, why is this being singled out as a separate category? To better understand AI-assisted design, we must note the various aspects in which the term “support” plays a key role.
In the context of the aforementioned generative design, we talk about so-called AI-driven design – a solution with a high degree of autonomy that can independently generate and optimize designs, acting as the primary “designer.”
But what about when we need more standardized parts based on good practices from our manufacturing plant but still want to save some time? AI-assisted design is a tool that supports the designer more, offering recommendations and helping automate design and optimize structures rather than executing the work for them. It relies on historical projects, material databases, 3D models, design documentation, and simulation results. Importantly, its effectiveness is enhanced by integration with product lifecycle management (PLM) systems.
Practical use of AI-Assisted Design
Let us analyze a simplified situation of how we could use the described tool to design a composite floor in a vehicle. The analysis should start by defining and inputting design requirements such as:
- dimensions,
- load,
- material properties,
- safety standards, etc.
Using AI-assisted design, we will get suggested materials based on previous designs or known composite properties.
We move on to the modeling stage once we preliminarily determine the composite materials. While creating the geometry, the tool can automatically recommend parameters such as composite layer thickness or fiber arrangement according to the predefined requirements. Then, AI-assisted design can help perform finite element analysis (FEA). The assistant would propose design optimization, suggesting geometry or material distribution changes to achieve the best simulation results.
Finally, the program can automatically generate technical documentation based on two sources. The first draws “inspiration” from drawings already in our system, while the second automatically generates a drawing based on the geometry and software functions.
In summary, such a tool is particularly effective in companies where projects are relatively repeatable – the so-called “same, but different” :).
Similar functions have been present in 3D software for years as single options but are now gaining importance thanks to rapidly developing AI and ML technology, which is becoming increasingly useful and creating a comprehensive chain of supported operations encapsulated in a single term referred to as “AI-Assisted Design.”
Summary
New tools and design methodologies based on artificial intelligence are emerging daily. Being open-minded and flexible in the face of dynamic change is highly valued in the work of an engineer. Even if your experience with AI support at work has been sporadic or negative, I strongly encourage you to test them again, as they are becoming more and more effective every day. We are not the only ones learning and evolving; AI is too 🙂
If you’re wondering whether it’s worth investing time in learning another CAD program, perhaps now is the time to examine more global changes in the approach to design.
Sources
- General Motors, Driving a lighter, more efficient future of automotive part design
- Engineering Applications of Artificial Intelligence in Mechanical Design and Optimization, Jozef Jenis, Jozef Ondriga
- Ford Service Content
- A quick introduction to the Creo Behavioral Modeling Extension
- Siemens adds intelligence-based design to Xcelerator portfolio with latest release of NX
Leave a comment